Machine learning for modelling and control of direct air capture systems
Find Similar History 12 Claim Ownership Request Data Change Add FavouriteTitle
CoPED ID
Status
Value
Start Date
End Date
Description
EPSRC : Stefan Radic Webster : EP/S022937/1
The placement project will investigate the use of machine learning for modelling and control of an offshore wind powered direct air capture (DAC) system. DAC remove carbon dioxide (CO2) from the atmosphere which is injected into deep-sea reservoirs and are a key negative emission technology, which are becoming increasingly vital as a climate change mitigation strategy to achieve the targets of the Paris Agreement. The problem of controlling DAC systems is difficult due to the complex operation and the intermittency of the wind power supply. A traditional approach for controlling DAC systems uses mathematical modelling, which is time consuming, requires specialist expert knowledge and is computationally expensive to execute. The project will use a data-driven approach to modelling and controlling DAC systems by drawing on the latest developments in machine learning such as deep learning and reinforcement leaning. The objective is to show the feasibility of machine learning in this setting and implement an adaptive, learning-based controller that maximises the CO2 capture rate.
University of Bristol | LEAD_ORG |
University of Victoria | PP_ORG |
Peter Flach | PI_PER |
Subjects by relevance
- Machine learning
- Climate changes
- Carbon dioxide
- Emissions
- Climate protection
- Modelling (representation)
- Wind energy
- Greenhouse gases
- Artificial intelligence
Extracted key phrases
- Direct air capture system
- DAC system
- Machine
- Control
- Wind power supply
- Mathematical modelling
- Stefan Radic Webster
- Co2 capture rate
- Deep learning
- Placement project
- Offshore wind
- Use
- Climate change mitigation strategy
- Key negative emission technology
- Traditional approach
Related Pages
UK Project Locations
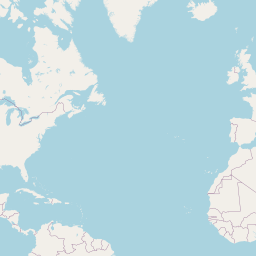
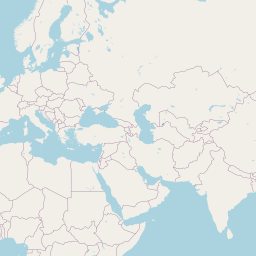
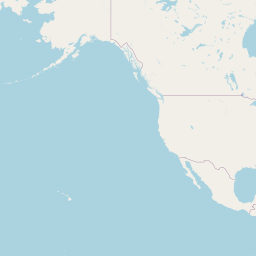
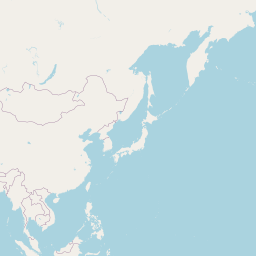



